A Class of Beta Second Kind Mixture Distributions
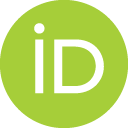
- DOI
- 10.2991/jsta.d.201229.001How to use a DOI?
- Keywords
- Methods of moments; Mixing distribution; Mixtured distribution
- Abstract
A class of mixture distributions have been derived which we call beta second kind mixtures of distributions. Various integral representations of beta functions can be obtained using these mixture beta distributions. Estimation of unknown parameters along with some characteristics of these distributions are also been found.
- Copyright
- © 2021 The Authors. Published by Atlantis Press B.V.
- Open Access
- This is an open access article distributed under the CC BY-NC 4.0 license (http://creativecommons.org/licenses/by-nc/4.0/).
1. INTRODUCTION
Mixture distribution [1,2] was first coined in 1894. A number of authors like Pearson [3], Rider [4], Blichke [5–8], Cohen [9], Chahine [10], Hasselblad [11], Day [12], Jewell [13], Roy et al. [14–16], Adnan et al. [17–26] defined mixtures of two distributions and studied various mixtured distributions which they called poisson mixture, binomial mixture, negative binomial mixture, chi-square mixture, erlang mixture, laplace mixture, pareto mixture, F mixture, weibull mixture and Maxwell mixture of distributions. Adnan et al. [27,28] also studied several properties of triple and folded Gamma mixture distributions.
2. PRELIMINARIES
A mixture distribution is a weighted average of probability distribution of positive weights that sum to one. The weights themselves constitutes a probability distribution. The mixing distribution
3. MAIN RESULTS
Here in this paper, we define the beta second kind mixtures of some well-known distributions such as normal, lognormal, gamma, exponential, beta second kind, rectangular, erlang, chi-square, t, and
Definition 3.1
A random variable X is said to have a beta second kind mixtured distribution if its probability density function is defined as
Definition 3.2
If X follows a beta second kind mixture of normal distribution with parameters p and q, then the density function is given by
The characteristic function and moments of the same distribution are presented in the theorem below.
Theorem 3.1.
If X has a beta second kind mixture of normal distributions with parameters
Remark
If
Definition 3.3
If a random variable X has the density function
Various moments of the distribution are given in the next theorem.
Theorem 3.2.
If X is a beta second kind mixture of lognormal variable with parameters
Definition 3.4
A random variable X having the density function
The characteristic function and moments are provided in the theorem below.
Theorem 3.3.
If X denotes a beta second kind mixture of gamma variate with parameters
Remark
If
Estimates of parameters by the method of moments: Let
Definition 3.5
A random variable X having the density function
Various characteristics of the above distribution are described in the following theorem.
Theorem 3.4.
If X follows beta second kind mixture of exponential distributions with parameters
Remark
If
Method of moments: If
Definition 3.6
If a random variable X has the density function
The characteristic function as well as the moments is stated in the following theorem.
Theorem 3.5.
If X has beta second kind mixture of erlang distributions with parameters
Remark
If
Estimating parameters: For a random sample
Definition 3.7
A random variable X having the density function
Different moments of the abovementioned distribution are expressed in the theorem below.
Theorem 3.6.
If X follows a beta second kind mixture of rectangular distribution with parameters
Remark
If
Definition 3.8
A random variable X having the density function
Different moments of the same distribution are provided in the following theorem.
Theorem 3.7.
If X follows beta second kind mixture of beta distribution of first kind with parameters
Remark
If we put
Definition 3.8
A random variable
Some characteristics of the distribution are represented in the theorem below.
Theorem 3.7.
If
Remark
Putting
Estimates of parameters: Let
Definition 3.9
If
The following theorem expresses here some of the properties of the distribution.
Theorem 3.8.
If
Remark
If
Definition 3.10
A random variable
The following theorem presents the characteristic function and moments of this distribution.
Theorem 3.9.
If
Then,
Remark
If
4. CONCLUSION
Various integral representations of beta functions can be found using the mixture beta distributions. These integrals are useful in finding mathematical and statistical properties of the various beta behavioral populations. A comparison among various features of the different beta second kind mixture distributions is shown in the tables of Appendix.
CONFLICTS OF INTEREST
Author has no conflicts of interest.
APPENDIX
A comparison among various features of the different beta second kind mixtured distributions is shown in the following Tables A1 and A2.
Sl. | Name of the Distribution | Probability Density Function |
Support | Parameters |
---|---|---|---|---|
1 | Beta 2nd kind mixture normal | |||
2 | Beta 2nd kind mixture lognormal | |||
3 | Beta 2nd kind mixture gamma | |||
4 | Beta 2nd kind mixture exponential | |||
5 | Beta 2nd kind mixture Erlang | |||
6 | Beta 2nd kind mixture rectangular | |||
7 | Beta 2nd kind mixture beta 1st kind | |||
8 | Beta 2nd kind mixture chi-square | |||
9 | Beta 2nd kind mixture t | |||
10 | Beta 2nd kind mixture F |
Comparison of density functions of different beta second kind mixture distributions.
Sl. | Name of the Distribution | Mean | Variance |
---|---|---|---|
1 | Beta 2nd kind mixture normal | ||
2 | Beta 2nd kind mixture lognormal | Can be obtained from Equation (7) | Can be obtained from Equation (7) |
3 | Beta 2nd kind mixture gamma | ||
4 | Beta 2nd kind mixture exponential | ||
5 | Beta 2nd kind mixture Erlang | ||
6 | Beta 2nd kind mixture rectangular | Can be achieved from Equation (22) | Can be achieved from Equation (22) |
7 | Beta 2nd kind mixture beta 1st kind | Equation (25) provides | Equation (25) provides |
8 | Beta 2nd kind mixture chi-square | ||
9 | Beta 2nd kind mixture t | ||
10 | Beta 2nd kind mixture F |
Comparison among first two moments of different beta second kind mixture distributions.
Comments If
REFERENCES
Cite this article
TY - JOUR AU - Mian Arif Shams Adnan AU - Humayun Kiser PY - 2021 DA - 2021/01/04 TI - A Class of Beta Second Kind Mixture Distributions JO - Journal of Statistical Theory and Applications SP - 518 EP - 525 VL - 19 IS - 4 SN - 2214-1766 UR - https://doi.org/10.2991/jsta.d.201229.001 DO - 10.2991/jsta.d.201229.001 ID - Adnan2021 ER -