Multivariate Escher Transformed Laplace Distribution and Its Generalization
- DOI
- 10.2991/jsta.d.200508.001How to use a DOI?
- Keywords
- Autoregressive processes; Generalized multivariate Esscher transformed Laplace distribution; Geometric multivariate Esscher transformed Laplace distribution
- Abstract
This paper we introduced a new distribution namely the multivariate Esscher transformed Laplace distribution. Various properties of the distribution are studied and the applications are discussed. Further we develop an autoregressive process with multivariate ETL marginal and study its properties. A Levy process based on this multivariate infinitely divisible distribution is known as Laplace motion, and its marginal distributions are multivariate generalized Esscher transformed Laplace distribution.
- Copyright
- © 2020 The Authors. Published by Atlantis Press B.V.
- Open Access
- This is an open access article distributed under the CC BY-NC 4.0 license (http://creativecommons.org/licenses/by-nc/4.0/).
1. INTRODUCTION
Esscher [1] introduced Esscher transformation for density approximations and later Daniels [2] and Barndorff-Nielsen [3] extended it as a general probabilistic method. Now this method is used as a means for creating a regular exponential family from a distribution whose cumulant generating function converges in the regular sense. Sebastian and Dais [4] introduced the Esscher transform of the classical Laplace distribution namely one parameter Esscher transformed Laplace (ETL) distribution and studied its various statistical properties viz infinite divisibility, geometric infinite divisibility, stability with respect to geometric summation, maximum entropy, finiteness of moments, simplicity, etc. Being heavy-tailed and asymmetric, this distribution is often used for modeling data related to various phenomena that range from image and speech recognition to ocean engineering. Some applications, extensions and generalizations of the one parameter ETL distribution is also introduced and studied (for details see, Dais and Sebastian [5,6], dais et al. [7] and Sebastian et al. [8]).
In this paper, we introduce two new distributions namely, multivariate ETL distribution and geometric multivariate ETL distribution. Autoregressive process with multivariate ETL distribution as marginal is also introduced. Generalizations of the multivariate ETL distribution and multivariate geometric ETL distribution are also considered and studied.
The probability density function and distribution function of the ETL distribution (
Graphs of the pdf of ETL(
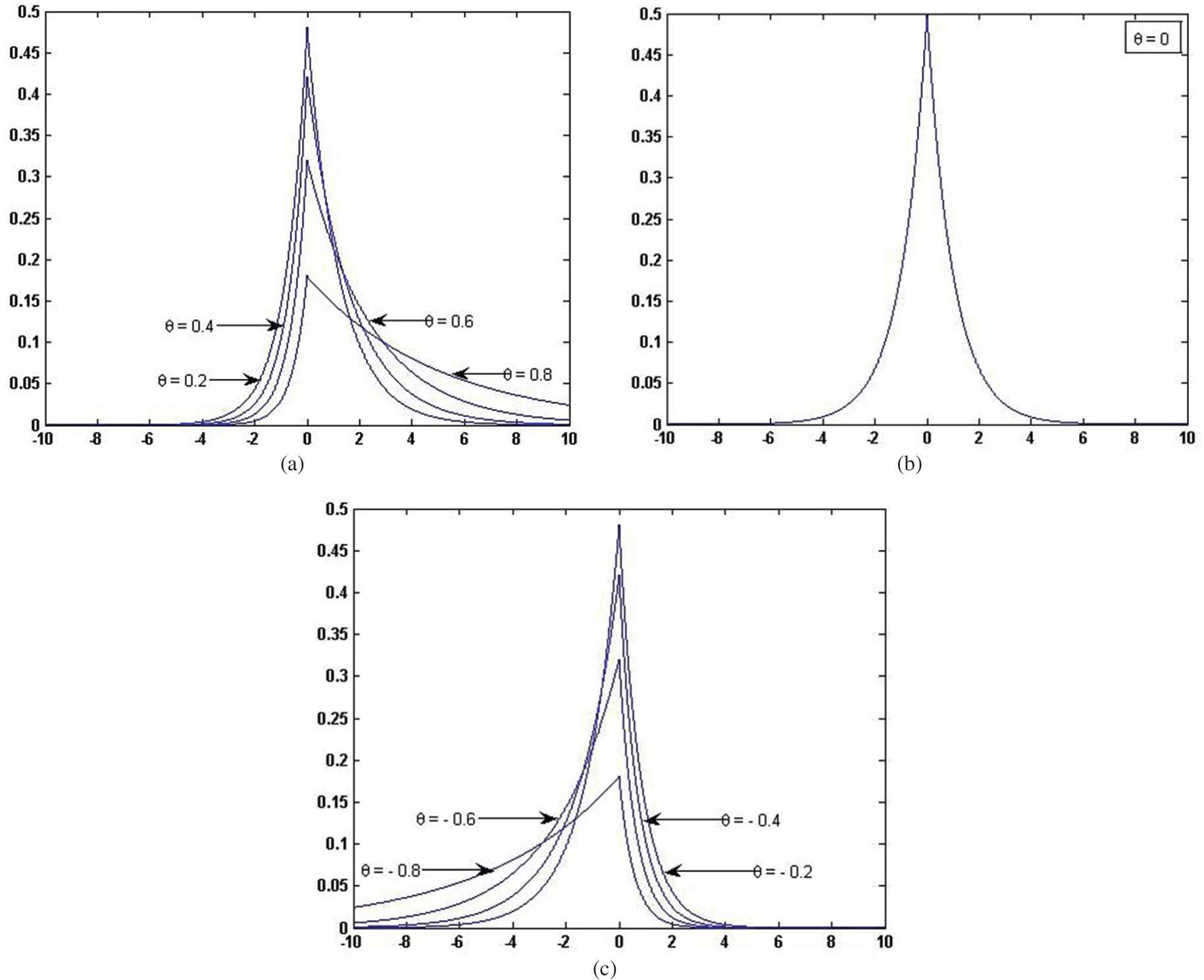
Densities of Esscher transformed Laplace distribution for (a)
Also the probability density function and distribution function of the location scale family of the ETL(
Graphs of the pdf of ETL(

Densities of Esscher transformed Laplace distribution for (a)
It is clear from the graph that the distribution is asymmetric and heavy-tailed. The ETL(
The remaining part of the paper is as follows: In Section 2, multivariate ETL distribution, its properties and the corresponding autoregressive processes are studied. Generalized multivariate ETL distribution is discussed in Section 3 and geometric multivariate ETL distribution and its generalization is considered in Section 4. Some applications of the distributions are discussed in Section 5.
2. MULTIVARIATE ETL DISTRIBUTION
In this section, we consider the multivariate extension of the ETL distribution. The characteristic function of the ETL distribution is
So the probability density function of
Since ETL distribution is the scale-location mixtures of normal distributions, if
If
2.1. Properties
Some important properties of the multivariate ETL distribution are discussed here.
P1. Infinite Divisibility
The characteristic function of the multivariate ETL distribution can be written in the form
P2. Stability
Let
2.2. Multivariate ETL Processes
Because of the wide applicability of time series models with non-Gaussian marginals in various fields such as biological, engineering, telecommunications, etc., many researchers are concentrating on introducing time series models with non-Gaussian univariate and multivariate marginals. For more reference see, Jose and Seethalekshmi [9], Alexandre Trindade et al. [10], Jayakumar and Kuttikrishanan [11], Dais et al. [7] and Asma Mustafa et al. [12].
In this section, we consider first order autoregressive model (linear additive) given by
Assuming stationarity, we have
So the innovation sequence
Theorem 2.1.
The multivariate ETL process is stationary with
Proof:
By method of induction, we can prove the result as follows:
Assume that
2.3. Distribution of Sums and Joint Distribution of ( X n , X n − 1 )
The distribution of sums
Then
By inverting
The joint distribution of
That is,
3. GENERALIZED MULTIVARIATE ETL DISTRIBUTION
In this section, we consider a generalization of the multivariate ETL (
Remark 3.1
If
Distributions on
Remark 3.2
A generalized multivariate ETL random variable admits mixture representation (8) where Z has a gamma distribution with the density
The density corresponding to (15) can be expressed in terms of Bessel function as
4. GEOMETRIC MULTIVARIATE ETL DISTRIBUTION AND ITS GENERALIZATION
From the recent related studies, we can see that geometric classes of distributions have wide variety of applications in the field of reliability, insurance mathematics, biological sciences and engineering. So we just find out the characteristic functions of both geometric multivariate ETLdistribution, denoted by Geometric
Theorem 4.1.
Let
Theorem 4.2.
Generalized geometric multivariate ETL distribution is the limit distribution of geometric sum of random vectors following
5. APPLICATIONS
In this article, we introduce some new asymmetric and heavy-tailed distributions, namely multivariate ETL distribution [
Similarly in Dais et al. [7], we consider the data sets, differences in flood levels per hour of Achenkovil river between two stations of Pathanamthitta district. Here also the multivariate distributions now developed can be used to study flood levels per hour of Achenkovil river between different stations in adjacent geographic zones so that the complexity of problems associated with water management faced by the State of Kerala due to the high variations in spatial and temporal rainfall can be made under control. Again in Dais George and Sebastian George [5], we model the file size distribution of Web server data with the ETL distribution and we know that many data sets related with Web servers (which are interrelated also) are asymmetric and heavy-tailed and can be modeled with ETL distribution. The geometric classes of distributions also have wide variety of applications in the field of reliability, insurance mathematics, biological sciences and engineering. As such all the newly introduced distributions are more useful and tactable.
CONFLICT OF INTEREST
No conflict of interest to declare.
REFERENCES
Cite this article
TY - JOUR AU - H Rimsha AU - Dais George PY - 2020 DA - 2020/07/29 TI - Multivariate Escher Transformed Laplace Distribution and Its Generalization JO - Journal of Statistical Theory and Applications SP - 325 EP - 331 VL - 19 IS - 2 SN - 2214-1766 UR - https://doi.org/10.2991/jsta.d.200508.001 DO - 10.2991/jsta.d.200508.001 ID - Rimsha2020 ER -