Risk Forecasting in the Light of Big Data
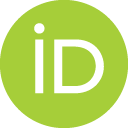
- DOI
- 10.2991/jracr.k.201230.001How to use a DOI?
- Keywords
- Big data; risk forecasting; systemic risk; predictive analytics; machine learning; security risk; sustainable development risk; financial risk
- Abstract
Life in modern society is increasingly connected by networks that link the world around us and create numerous exciting opportunities, new services and advantages for humanity. Yet concurrently, these underpinning networks have provided routes by which potentially dangerous and harmful incidents can propagate quickly and worldwide. This complexity poses a considerable challenge for risk analysis and forecasting. Conventional methods of risk analysis tend to underestimate the probability and impact of risks (e.g. pandemics, financial collapses, terrorist attacks), as sometimes the existence of independent observations is wrongly assumed and cascading errors that can occur in complex systems are not considered. The purpose of this article is to assess critically the potential of big data to profoundly change the current capability for risk forecasting in diverse areas and the assertion that big data leads to better risk predictions. In particular, the focus is on big data implications for risk forecasting in the areas of economic and financial risks, environmental and sustainable development risks, and public and national security risks. The article concludes that big data and predictive analytics offer substantial opportunities for improving risk forecasting but may not replace the significance of appropriate assumptions, adequate data quality and continuous validation.
- Copyright
- © 2021 The Authors. Published by Atlantis Press B.V.
- Open Access
- This is an open access article distributed under the CC BY-NC 4.0 license (http://creativecommons.org/licenses/by-nc/4.0/).
1. INTRODUCTION
Society is increasingly connected through networks that link the world around us and facilitate a global exchange of people, commodities, capital, knowledge and ideas, create numerous exciting opportunities, new services and advantages for humanity. Yet concurrently, the underpinning networks have provided routes by which potentially dangerous and harmful incidents can propagate quickly and worldwide. This complexity poses a considerable challenge for risk analysis and forecasting. Conventional methods of risk analysis tend to underestimate the probability and impact of risks (e.g. pandemics, financial collapses, terrorist attacks), as sometimes the existence of independent observations is wrongly assumed and cascading errors that can occur in complex systems are not considered. Big data, machine learning, and predictive analytics offer new opportunities for understanding and managing risks in complex environments and there is a widespread belief that big data can aid in improving risk forecasts [1–3]. Many institutions are already adopting innovative ways to use big data analytical methods to improve their risk assessment processes and to predict risks from economic, social, or environmental data [4–6]. This article will discuss the application of big data and predictive analytics for risk forecasting in general and in the specific context of economic and financial risks, environmental and sustainable development risks, and public and national security risks. The article will begin by explaining the concepts of risks and big data and will then address the potential promises and pitfalls of using big data for risk analysis and forecasting. Following this, common types of analytical methods for big data risk forecasting are identified and a general framework for their use is outlined. The last section of this article discusses the application of big data analytics in the abovementioned risk domains.
Risks exist in every area of life, and in both the business world and the public administration, decisions have to be made constantly, the outcome of which is uncertain. Understanding the uncertainty, however, may help us make better decisions. A risk in this context can be defined as a random event that may possibly occur and, if it does occur, would have a negative impact on the objectives of the entity [7]. Different disciplines have different ways of classifying risks. A common classification consists of the division into the three categories of: ‘known knowns’, ‘known unknowns’, and ‘unknown unknowns’, corresponding to different levels of uncertainty [8]. The term “unknown unknowns” was brought to the fore by US Secretary of Defense Donald Rumsfeld at a press conference in 2002 when he addressed the lack of evidence for a link between the Iraqi government and the supply of weapons of mass destruction to terrorist groups. The term has, however, been used long before this [9]. In a risk context, the idea of unknown unknowns intuitively grasps the fact that the events actually occurring are not covered by the events identified in the risk assessment.
The risks that threaten modern societies compose a complex network that often underlies crisis events [10]. So far, however, little is known about how risk materializations in different domains influence each other. Modern societies are highly dependent on the reliable functioning of systems that are interconnected in explicit or implicit ways [11]. Whilst the increase in interconnection between various infrastructural systems can lead to a higher level of service efficiency, it also makes the involved systems susceptible as a whole to cascading failures [2,12]. Such cases of cascades of failures have been studied in model networks in general and in the context of transport systems, in financial institutions and within ecological systems in particular. Besides the risk of cascade failures occurring within a specific domain, there are other risks arising from the interconnection between systems in different domains. In fact, the key thesis behind many collapses of society in modern history is that there is a cascade of different risks that are unfolding, and hence there is a particular need to quantify the dynamics of large-scale risk materialization that looms in this globally interconnected web. In this context, the predictive analysis of big data offers enormous opportunities for new insights, especially about networks, spatial and temporal dynamics, for the understanding of human systems on the systemic level and for the detection of interactions and nonlinearities in the relationships between variables [13].
2. IMPLICATIONS OF BIG DATA FOR RISK FORECASTING
Big data technology is seen as the digital-age equivalent of the telescope or microscope [14]. New repositories for powerful data are emerging from social media websites, search engines and other sources, which accumulate vast amounts of information every second. International Data Corporation predicts that the global datasphere will grow from 33 zettabytes (ZB) in 2018 to 175 ZB by 2025 [15]. While conceptions of big data have been around since the 1990s, the term only reached a higher level of relevance in technology and in the public perception during the last 15 years. The term big data is used to describe exceptionally large datasets that can only be analyzed by computation, either separately or in combination with other sets of data to uncover previously unrecognized patterns, trends and associations. The uniqueness of big data approaches lies in the fact that they offer the ability to collect and analyze data in a range and depth that would otherwise be difficult to achieve [16,17]. There are typically three characteristics associated with big data, often referred to as the three Vs: volume, variety, and velocity [17–19]. In this context, (1) Volume refers to the processing of ever larger amounts of data (e.g. terabyte, petabyte or larger); (2) Variety refers to the diversity of the generated data, which may come from a variety of different sources and is generally one of three types: structured, semi structured and unstructured data. The variety in data types frequently requires distinct processing capabilities and specialist algorithms; (3) Velocity refers to the speed at which data is generated, processed and analyzed.
Several countries and organizations have initiated various complex and large-scale projects to use big data, such as the European Human Brain Project [20] or CSIRO’s Australian Square Kilometre Array Pathfinder [21], demonstrating that big data is useful for more than just relatively small problems, viz. well-structured cases characterized by repeated evaluation of predictions [22]. Furthermore, data mining and machine learning have emerged as powerful tools for big data forecasting to exploit the power of unstructured data and to extract new knowledge and identify significant patterns and correlations hidden in the data [23]. These two developments together have recently sparked an all-encompassing enthusiasm based on the idea that large data will lead to much better forecasts in all domains, from scientific discovery to medical, financial, commercial and political applications. Recently, it even led to the idea that for the domain of predictive analysis, some kind of master algorithm could possibly be developed one day [24]. However, as some simple examples such as weather forecasting show, the best forecasts generally arise from a reasonable compromise between modelling and quantitative analysis, where conceptual insight counts as much as the amount of data [25]. The capability of making predictions from the data alone may be theoretically possible, but in practice it is often unachievable, even in the face of a clear situation where one has perfectly accurate information about the system. Hence, big data does not make the importance of data quality and modelling assumptions obsolete.
While big data can be crucial for understanding risks in interdependent systems, particularly in the field of disaster modelling, and can also improve the accuracy of traditional risk assessment techniques, risk analysis is clearly not just a computational challenge that can be solved by more data. Ultimately, for an accurate prediction, it must be possible to understand the risk analysis in order to moderate the occurrence and impact of negative outcomes. The difficulties of using big data for risk analysis can be illustrated with the example of Google Flu Trends (GFT). The Google flu tracking system is frequently cited as a negative example for the usage of big data forecasting, although the erroneous forecasts in this particular case were arguably caused by the algorithm dynamics that influenced Google’s search algorithm and the changes that Google itself had made to the search algorithm [26]. GFT has persistently overestimated flu prevalence, predicting more than twice the percentage of consultations for influenza-like illnesses than the US Centers for Disease Control and Prevention, which have based their estimates on surveillance reporting from labs located throughout the United States. The mistakes made in the conceptual design of GFT have often been described as big data hubris in a general criticism of the tendency to see big data not as a supplement but as a replacement for traditional data collections and their analysis.
3. TYPES OF ANALYTICAL METHODS FOR BIG DATA RISK FORECASTING
The ability to conduct predictive analysis based on large volumes of data is one of the interesting opportunities arising from the spread of large data architectures. An increase in computing power and memory, along with improved algorithms and a better knowledge of their application now enable us to build models from very large data sets [23]. In essence, the techniques of predictive analysis, which attempt to discover patterns and capture relationships in data, can be divided into two groups: while some of the techniques are aimed at discovering historical patterns in the outcome variables and extrapolating them into the future, others attempt to capture and use the interdependencies between the outcome variables and the explanatory variables for predictions. Among the techniques commonly used nowadays for predicting large amounts of data are logistic and linear regression models, decision trees, perceptrons, artificial neural networks, association rules, k-nearest neighbors, latent semantic analysis, Naive Bayes classifier and random forests [27]. Further innovations in techniques for big data forecasting can be expected in the near future, with real-time analysis in particular, due to the growth of location-based social media and mobile applications, likely to become a promising field of research and development [17].
Notwithstanding the hype surrounding the various methods for big data forecasting, the application of such analytics is still a labor-intensive process [28]. Recent solutions for the analysis of big data are often based on proprietary applications or on general purpose software systems. Typically, organizations require significant efforts to adapt such solutions to their individual needs, which may include integrating different data sources and implementing the software on the organization’s hardware. Today, a number of advanced technologies for analyzing large amounts of data (e.g. BigQuery, Hadoop, MapReduce, WibiData, and Skytree) are commercially available that enable the generation of insights to improve organizational strategies and decision-making processes.
The remainder of the article will focus on big data implications for risk forecasting in the areas of economic and financial risks, environmental and sustainable development risks, and public and national security risks.
4. PUBLIC AND NATIONAL SECURITY RISKS FORECASTING
Intelligence agencies and law enforcement authorities around the world are confronted with an increasingly complex spectrum of threats from a variety of different origins and backgrounds: national governments, groups and individuals with widely differing motivations and a growing arsenal of approaches. Terrorism, crime and other security challenges can only be adequately dealt with if reliable information is available in good time and successful operations must be able to use information from a variety of sources, such as human intelligence, signals intelligence and open source intelligence. Big data promises to uncover hitherto undetected security-related patterns and to reveal unexpected hidden knowledge that may hold the key to the prevention of future crimes or acts of terrorism [5,29,30]. Public authorities therefore have invested substantially in big data collection platforms and technologies for gathering and analyzing information [31].
By increasingly adopting data processing methodologies for forecasting purposes, security experts have been influenced by similar big-data applications in the corporate world. In intelligence, counterterrorism, policing and peacekeeping, operations have been transformed by the capabilities of big data and predictive analysis to detect unexpected security-related patterns and identify potential threats [32,33]. Predictive analysis with big data offers security experts the promise of safeguarding the future by anticipating the next terrorist attack and detecting potential crimes before they are committed. Consequently, predictive analysis is used for the purpose of forward-looking decision-making to respond to the growing range of security issues, from terrorism and crime to natural disasters and poverty [34–36]. The shift from past data analysis to the prediction of future events is a central claim of big data analytics. This increased focus on the future will presumably reinforce the division between surveillance on the one hand and case history on the other, and the intense pursuit of pattern discovery will probably warrant a significant expansion of data access [5,37].
In the context of policing, the forecasting promise of big data has received considerable public recognition, making predictive policing one of the latest expressions of a “big data revolution” for security operations [34]. Predictive policing, defined as the application of analytical techniques, and in particular quantitative techniques, to help in the identification of promising targets for police interventions and to prevent or solve crimes, can offer a number of distinct advantages to law enforcement agencies [38]. Police authorities in the United States and Europe have recently purchased commercially available software (e.g. PredPol, Keycrime) for predicting crimes [25].
The integration of artificial intelligence (AI), machine learning and large databases in counterterrorism and crime-fighting has been applied to a variety of intelligence and operational missions, such as the determination of the structure of terrorist networks and criminal organizations, the localization of high value targets, etc. [29]. The use of AI and big data analytics for intelligence objectives is a novel approach to information extraction that transforms each stage of the intelligence process, starting with the gathering and analysis of information, through to the development of the intelligence picture and the conversion of the information into operational measures [39].
The use of forecasting with big data in the intelligence and counter-terrorism arena has, not least because of the Edward Snowdon revelations, sparked intense controversy between advocates of the pursuit and expansion of the deployment of this technology and its critics. Advocates of the use of big data and AI in the intelligence domain argue that their effectiveness in this area has long been proven and that many authorities around the world have used them and have experienced great success [32]. These proponents argue that almost everyone today has a digital footprint that can be traced and analyzed, and therefore much data can be collected through the use of mobile phones, computer systems, apps, social networks, electronic communications, and many other technologies [34]. Arguments opposing the use of big data analysis can be divided into three categories: generic arguments reflecting concerns about the growing use of big data and AI and the impact of these developments on our modern society as a whole; use-related claims that it is impossible to exploit big data in an effective way in terrorism and crime prevention; and ethical considerations, which suggest that the possibility of harm to innocent civilians caused by the use of big data prediction in intelligence and crime prevention should preclude the use of this technology [29]. The traditionally fragile equilibrium between effectiveness in the fight against crime and terrorism and the liberal democratic principles of society is becoming even more critical when AI and big data analytics-based countermeasures are employed. The integration of AI and big data for intelligence purposes has proven to be effective in detecting terrorists and criminal activity and has helped to thwart terrorist attacks [29,40]. However, this technological approach is more advanced than the current regulatory framework and consequently poses a significant risk of violating human rights and privacy [41–43]. Moral and ethical issues arising from the use of these technologies include, on the one hand, the fact that the rights of the individual, in particular the privacy of citizens, freedom of speech and opinion, could be violated. If the government can classify certain utterances as suspicious and if it is able to monitor statements made by all citizens on social networks as well as their daily behavior as expressed in various databases, it should be considered that the government can misuse this information. On the other hand, the inherent error margin of this intelligence gathering approach is liable to subvert the legal rights of suspected persons to conduct fair investigations and legal proceedings, causing them irreparable harm and possibly even putting their lives at risk. Screening for perpetrators is a difficult methodological problem, whether it is manual profiling by experts or AI and big data-based automatic profiling of individuals. The problem arises from the low prevalence of perpetrators in the population, known as “finding the needle in the haystack”, which can lead to a high rate of false positive results [44].
4.1. Big Data for Predicting Risks of Political Instability
Forecasting with big data has become increasingly important, not only in the areas of counterterrorism and crime-fighting, as described above, but also in predicting risks of political instability. Companies, governments and international organizations have good reasons to make efforts to anticipate such risks. Businesses want to know the risks of investing in volatile sectors, while governments must focus their policies and foreign aid on alleviating human suffering and economic collapse [45]. The capability to successfully predict political instability – from the risk of societal unrest, riots and protests to civil wars and interstate wars, violent coups, genocides or state collapse – would profoundly alter the ability of nations to proactively respond to global instability and intervene before unrest escalates into conflict, or to provide the means to improve preventive measures.
One of the most important means of feeding modelling approaches in the field of conflict forecasting is the event database. Political event data are recordings of interactions between political actors using a shared set of codes for both actors and actions, which allow for an aggregated analysis of political behavior [4]. Databases of such events are essentially recordings of both material interactions between political entities and verbal expressions, which are used to search for temporal patterns in the chronological order and intensity of the records [46].
Essentially, conflict forecasting approaches have assumed three different forms: (1) individual experts who summarize available information and form a judgement, (2) collections of such experts who are brought together to form a consensus view, and (3) data-driven computer models that use patterns of past actions to predict future physical behavior. Recent progress in computational methods, and in particular in text analysis, has enabled automation of the data-drive approach significantly, thus allowing far more comprehensive sources of real-time information to be analyzed, thereby facilitating the transition from structural to short-term registration of tensions and other conflict characteristics [47].
Event databases like Defense Advanced Research Projects Agency (DARPA)’s Integrated Conflict Early Warning System [48] and the European media monitor [49] are currently among the most prominent of these automated and integrated systems to monitor, assess, and forecast national, sub-national, and internal crises. Both compile extensive real-time archives of incidents of physical disturbances in countries of interest and compile regular reports that summarize the main emerging spatiotemporal dynamics. Machine-readable documents on political issues, including press releases, announcements, presentations, press briefings, and intelligence reports, the volume of which is constantly increasing, have become the basis of many policy analyses [4]. The expansion of machine-readable content is being driven by the increasing online publication of established media sources such as Agence France-Presse and Reuters, the growth of a number of locally based agencies that regularly report in English and publish these reports online, and the advent of international media sources including BBC Monitoring, Al-Jazeera, XinhuaNet and AllAfrica.
The constantly rising availability of these types of documents offers both opportunities and challenges for risk forecasting of political instability [4,47,50]. While these changes in the global news media industry, as well as worldwide internet accessibility, provide significant potential for novel applications, the inherent process of generating event data, which is not static but rather very dynamic, also creates a number of technical challenges and requires frequent, if not continuous, validation activities from users. An advantage of machine-encoded event data as a viable alternative to human experts is the significantly lower cost of machine coding, for which there is virtually no marginal costs in a well-developed system if the texts are collected automatically. Additionally, it is much easier and more cost-effective to modify an existing machine-coding solution than to instruct human experts in an existing protocol [4].
In addition to news, information from social media is also attracting increasing attention, the advantage of which is both the considerable amount of data and the very nature of turning every user into a potential reporter, thus potentially extending the range of events covered considerably and facilitating real-time coverage of a wide range of events [47,51]. However, their use poses considerable challenges, as social media diffuses information and mobilizes people, and most content, such as Twitter content, is either not relevant for the purposes of conflict prediction or has been deliberately implanted with false information for manipulation [47,52].
5. ENVIRONMENTAL AND SUSTAINABLE DEVELOPMENT RISKS FORECASTING
Environmental risk forecasting contains many inherent uncertainties due to factors that go beyond the scope of ecology (e.g. demographic change, climate change, governance measures), unknown reactions in interconnected socio-ecological systems and unforeseeable human actions. The importance of estimating future environmental risks has increased in recent years as the pace of change processes has been accelerating and the level of uncertainties has risen [53]. With big data analytics tools, there is now a variety of novel ways to capture complex ecosystem interrelationships and provide the information needed to conduct environmental risk forecasting on a larger scale [54]. The following sections present a brief summary of the impact of big data analytics in the forecasting of climate risks and sustainable development risks.
5.1. Big Data Impact on Climate Risk Forecasting
Climate change is a major challenge for society, particularly in terms of its capacity to take individual and collective decisions that will enable appropriate responses to address it [55]. In many respects, it differs from other environmental problems facing modern civilization in its time scale and in its complex relationship between human activities, the embedded societal structures and interactions that are emerging between different environmental systems [56]. Climate change is leading to cascade-like risks in technical installations, ecological systems, the economy, and society, all of which are often interlinked and create the conditions for irreversible and unwanted exceeding of threshold values at various levels [57]. Forecasting climate risks across sectors and in a way that is meaningful to decision-makers thus represents a major scientific challenge. Big data analytics is seen as very promising in terms of predicting risks associated with climate change [22,58,59].
The Intergovernmental Panel on Climate Change has described the benefits of a risk-based approach to better understand both the dynamic interactions of spatial and temporal determinants leading to specific impacts of climate change, and the role of adaptation initiatives in managing corresponding risks [55]. The most fundamental components for the analysis and prediction of climate risks are verifiable, up-to-date and comparable data and relevant modelling. Conventional approaches to risk forecasting and assessment are challenged by the substantial temporal and geospatial dynamics of climate change, by the enhancement of risks of certain societal settings, and by the interaction of several risk factors. However, today big data from climate model simulations is increasingly being used to predict future trends in climate change and to assess the associated risks [59,60]. There are various ways in which big data elements could contribute to improving the modelling of climate risks and impacts. New forms of data could be useful for the calibration of risk models and crowdsourcing and crowdsensing data collected for a specific purpose could be useful, as the assumption of constancy can be justified by reference to the user base [22]. To manage their responses, stakeholders and policy makers need to forecast the potential local risk impacts of climate change at the county-to-city level. Part of this information could be derived by combining fine-grained climate risk assessments with AI-based big data analytics of weather extremes, property damage, health impairment and other variables [61]. A number of big data analytics-based tools for screening climate risks are currently being developed, including the World Bank’s “Climate and Disaster Risk Screening Tool” [62], and many institutions are using them to better understand climate risk in their decision-making.
The primary challenge in forecasting the risks of global climate change is clearly the complexity and myriad of interacting factors. Each incremental change in greenhouse emissions and temperature gives rise to different responses in climatological, ecological, hydrological and other biophysical systems, varying from short term impacts on primary productivity to longer term effects such as rising sea levels, degradation or land formation, whereby the coupling of systems can lead to reactions that affect other systems, including feedback effects on climate [56]. Some recent studies emphasize the changing nature of the three components of risk (hazard, exposure and vulnerability) and point to the need for the development of coherent guidance on strategies and methodologies that better account for the dynamic nature of the individual risk components and their interaction [63]. This is particularly important since climate risks are not only a function of physical processes and shifting characteristics of climate systems but are also shaped by complex interactions with socio-economic drivers that can change and evolve within the macroscale conditions and may also change norms and values. With the driving forces and physical consequences of climate change being better known, researchers are increasingly turning their focus on analyzing these socio-economic drivers of climate change. Big-data elements could become useful in this research field, as there are no well-proven universal theoretical concepts for such target systems [22].
5.2. Risk Indicators for Sustainable Development Risks Forecasting
Big data for development, which identifies the potential of big data analytics to produce practical information that can be used to improve global development, e.g. by analyzing and forecasting sustainable development risks, could complement established methods of handling sustainable development data by opening up new perspectives on problems and deepening and accelerating analysis [64,65]. There is a growing public understanding of global risks associated with environmental disasters, pollution, land degradation, poverty, food security, migration flows, and levels of violence and conflict. To quantify these risks and to support governance worldwide, a plethora of performance indicators and databases has been introduced in recent times [66]. An important driving force behind this development is related to the monitoring of the 17 Sustainable Development Goals (SDGs) and the United Nations’ call for a “data revolution for sustainable development” [67,68]. The risks to sustainable development are manifold and are shaped by the interactions between a variety of socio-economic factors and the changing physical environment. SDGs represent an unprecedented effort towards global sustainable development, the complexity of which makes the use of relevant risk indicators reasonable.
Currently, a multitude of organizations such as national and international institutions, universities, think tanks, investment organizations, journals, and reinsurance companies are publishing global risk indicators [66]. Such indicators are crucial to many sustainability initiatives because they are a useful tool for generating knowledge on complex issues, facilitating informed decision making and allow for effective communication between experts and non-experts as well as invaluable awareness raising on specific issues [69]. For the compilation of risk indicators, data quality is of great importance, especially considering the fact that this data can significantly influence the outcome of policies. Big data is expected to have the potential to support addressing development challenges and meeting the requirements for the development of SDG risk indicators [70]. Although risk indicators have been used in the past to forecast risks to socio-economic developments, in some cases the amount of data available allows big data to provide better predictions and improve forecasting accuracy by complementing existing statistical series with more granular, higher-frequency data.
6. ECONOMIC AND FINANCIAL RISKS FORECASTING
Technological advances have transformed economies and financial markets into increasingly more complex and dynamic systems, with market participants becoming ever more interconnected, transactions managed on timescales in the sub-millisecond range and masses of data generated, stored and processed [12,71]. These developments present new challenges and opportunities with regard to the understanding and management of risks in financial systems [72,73]. Part of this is the question of how we can leverage big data to create more accurate analytical methods for forecasting bubbles and collapses in economic and financial systems.
The global financial crisis of 2008 has prompted a large number of regulatory changes, but little progress has been made in providing early information on the vulnerabilities and risks of banks [74]. Therefore, building accurate scenario models of future financial risks and vulnerabilities is essential for economists, business leaders, private and institutional investors and policy makers to be able to make a realistic assessment of future economic developments and their implications, and to be prepared to respond appropriately. As analysis of the 2008 financial crisis has suggested, conventional models based on standard economic and financial estimates were not entirely satisfactory [6]. Forecasting banking distress is an important issue, with many efforts focused on identifying risk accumulation at an early stage, currently often using aggregate accounting data to measure imbalances. However, despite their rich information content, accounting data pose great challenges due to the low reporting frequency and long publication cycles. A key problem with traditional models is that they are usually unable to take into account the psychological responses of financial investors to certain incidents and disclosures. Market participants make predictions about the future development of share prices and other investment opportunities, which could be influenced by their mood or by inconsistent expectations, and the neglect of the entirety of these emotional behaviors could greatly distort the predictions of the models and lead to significant negative consequences, up to system-wide financial crises.
Most recent research that applies a form of text-based sentiment analysis to investigate the state of the economy or financial markets is using either news or social media generated data. The spread of the Internet and social media has created a huge amount of new data containing potentially revealing information about the sentiments, opinions, expectations and fears of its users. A better understanding of behavior in financial markets is expected to provide a more solid basis for political and economic decision-making and support risk management strategies [3,6,75]. So far, however, the analysis of social media data has mostly provided short-term indications that are of limited use for fundamental analysis. Such evidence is most useful for explaining events in retrospect rather than for making predictions. Nevertheless, social media analysis is an area that is considered to have great potential for future exploration and research [1]. An algorithmic analysis of sentiment trends in large volumes of financial news documents was used, for instance, by Nyman et al. to assess how narratives and moods play a role in influencing developments in the financial system [3,76]. According to Nyman et al., in their study, changes in emotional content in market narratives are highly correlated across data sources.
Many financial institutions are currently exploring innovative ways of using big data analysis to improve their internal risk assessment systems [77]. The development of better models identifying high-risk areas could improve the tools available to regulators for early detection of potential financial crises. An effort that would serve all stakeholders and could significantly increase efficiency would be to develop innovative ways to facilitate the management and exchange of data within the financial industry and with both academic researchers and national regulatory authorities. A corresponding research and development approach based on blockchain technology, where data is encrypted by breaking it down into blocks that are distributed to computer nodes, is currently being pursued by Massachusetts Institute of Technology (MIT) researchers [1]. Such a solution could have the potential to become a trusted tool for financial industry stakeholders for establishing new aggregated risk metrics and for understanding systemic risks better, because if financial institutions are confident, they can exchange data without revealing proprietary secrets.
7. CONCLUSION
Big data offers substantial opportunities for improving risk forecasting, but may not replace the significance of appropriate assumptions, adequate data quality and continuous validation [2,78,79]. Although there are different understandings as to whether or not the main methods of risk analysis for large amounts of data are similar to conventional methods, it is widely considered that the availability of big data allows novel risk analysis. Big data and predictive analytics cannot provide a sure-fire method for identifying all critical problems before they occur, but big data-driven development of more accurate early-warning indicators and ways to monitor patterns, are approaches that are more likely to avert an imminent risk and are a worthwhile effort for a range of different fields of application [1,10,80]. However, in order to derive the most benefit from it, the resulting advances at the interface of machine learning, statistics, and AI must be linked to an appropriate methodological basis, especially with regard to the possible ethical, social and legal consequences of a possible misuse of the application of such methods [5].
CONFLICTS OF INTEREST
The author declares no conflicts of interest.
REFERENCES
Cite this article
TY - JOUR AU - Roman Kernchen PY - 2021 DA - 2021/01/21 TI - Risk Forecasting in the Light of Big Data JO - Journal of Risk Analysis and Crisis Response SP - 160 EP - 167 VL - 10 IS - 4 SN - 2210-8505 UR - https://doi.org/10.2991/jracr.k.201230.001 DO - 10.2991/jracr.k.201230.001 ID - Kernchen2021 ER -