Deep Learning–Based CT-to-CBCT Deformable Image Registration for Autosegmentation in Head and Neck Adaptive Radiation Therapy
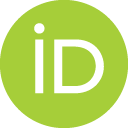
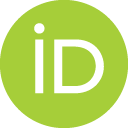
- DOI
- 10.2991/jaims.d.210527.001How to use a DOI?
- Keywords
- Deep learning; Deformable image registration; Segmentation; CBCT
- Abstract
The purpose of this study is to develop a deep learning–based method that can automatically generate segmentations on cone-beam computed tomography (CBCT) for head and neck online adaptive radiation therapy (ART), where expert-drawn contours in planning CT (pCT) images serve as prior knowledge. Because of the many artifacts and truncations that characterize CBCT, we propose to utilize a learning-based deformable image registration method and contour propagation to get updated contours on CBCT. Our method takes CBCT and pCT as inputs, and it outputs a deformation vector field and synthetic CT (sCT) simultaneously by jointly training a CycleGAN model and 5-cascaded Voxelmorph model. The CycleGAN generates the sCT from CBCT, while the 5-cascaded Voxelmorph warps the pCT to the sCT's anatomy. We compared the segmentation results to Elastix, Voxelmorph and 5-cascaded Voxelmorph models on 18 structures including target and organs-at-risk. Our proposed method achieved an average Dice similarity coefficient of 0.83 ± 0.09 and an average 95% Hausdorff distance of 2.01 ± 1.81 mm. Our method showed better accuracy than Voxelmorph and 5-cascaded Voxelmorph and comparable accuracy to Elastix, but with much higher efficiency. The proposed method can rapidly and simultaneously generate sCT with correct CT numbers and propagate contours from pCT to CBCT for online ART replanning.
- Copyright
- © 2021 The Authors. Published by Atlantis Press B.V.
- Open Access
- This is an open access article distributed under the CC BY-NC 4.0 license (http://creativecommons.org/licenses/by-nc/4.0/).
Download article (PDF)
View full text (HTML)
Cite this article
TY - JOUR AU - Xiao Liang AU - Howard Morgan AU - Dan Nguyen AU - Steve Jiang PY - 2021 DA - 2021/06/10 TI - Deep Learning–Based CT-to-CBCT Deformable Image Registration for Autosegmentation in Head and Neck Adaptive Radiation Therapy JO - Journal of Artificial Intelligence for Medical Sciences SP - 62 EP - 75 VL - 2 IS - 1-2 SN - 2666-1470 UR - https://doi.org/10.2991/jaims.d.210527.001 DO - 10.2991/jaims.d.210527.001 ID - Liang2021 ER -