Deep High-Resolution Network for Low-Dose X-Ray CT Denoising
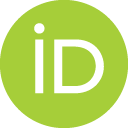
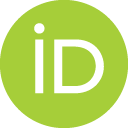
- DOI
- 10.2991/jaims.d.210428.001How to use a DOI?
- Keywords
- Low-dose CT; Deep learning; Denoise
- Abstract
Low-dose computed tomography (LDCT) is clinically desirable because it reduces the radiation dose to patients. However, the quality of LDCT images is often suboptimal because of the inevitable strong quantum noise. Because of their unprecedented success in computer vision, deep learning (DL)-based techniques have been used for LDCT denoising. Despite DL models' promising ability to remove noise, researchers have observed that the resolution of DL-denoised images is compromised, which decreases their clinical value. To mitigate this problem, in this work, we developed a more effective denoiser by introducing a high-resolution network (HRNet). HRNet consists of multiple branches of subnetworks that extract multiscale features that are fused together later, which substantially enhances the quality of the generated features and improves denoising performance. Experimental results demonstrated that the introduced HRNet-based denoiser outperformed the benchmarked U-Net–based denoiser, as it provided superior image resolution preservation and comparable, if not better, noise suppression. Quantitative evaluation in terms of root-mean-squared errors (RMSEs)/structure similarity index (SSIM) showed that the HRNet-based denoiser improve these values from 113.80/0.550 (LDCT) to 55.24/0.745 (HRNet), which outperformed the 59.87/0.712 for the U-Net–based denoiser.
- Copyright
- © 2021 The Authors. Published by Atlantis Press B.V.
- Open Access
- This is an open access article distributed under the CC BY-NC 4.0 license (http://creativecommons.org/licenses/by-nc/4.0/).
Download article (PDF)
View full text (HTML)
Cite this article
TY - JOUR AU - Ti Bai AU - Dan Nguyen AU - Biling Wang AU - Steve Jiang PY - 2021 DA - 2021/05/03 TI - Deep High-Resolution Network for Low-Dose X-Ray CT Denoising JO - Journal of Artificial Intelligence for Medical Sciences SP - 33 EP - 43 VL - 2 IS - 1-2 SN - 2666-1470 UR - https://doi.org/10.2991/jaims.d.210428.001 DO - 10.2991/jaims.d.210428.001 ID - Bai2021 ER -