Multi-Scale Person Localization With Multi-Stage Deep Sequential Framework
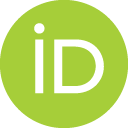
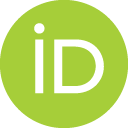
- DOI
- 10.2991/ijcis.d.210326.001How to use a DOI?
- Keywords
- Scale estimation; Deep learning; Head detection; Crowd analysis
- Abstract
Person detection in real videos and images is a classical research problem in computer vision. Person detection is a nontrivial problem that offers many challenges due to several nuisances that commonly observed in natural videos. Among these, scale is the main challenging problem in various object detection tasks. To solve the scale problem, we propose a framework that estimates the scales of person’s heads, as we argue that head is the only visible part in complex scenes. we propose a head detection framework that explicitly handles head scales. The framework consists of two sequential networks: (1) scale estimation network (SENet) and (2) head detection network. SENet predicts the distribution of scales from the input image in the form of histogram. Then the scale histogram adjust anchor scale set of region proposal network that generates object proposals. These objects proposals are then classified into two classes, that is, head and background by the detection network. We evaluate proposed framework on three challenging benchmark datasets. Experiment results show that proposed framework achieves state-of-the-art performance.
- Copyright
- © 2021 The Authors. Published by Atlantis Press B.V.
- Open Access
- This is an open access article distributed under the CC BY-NC 4.0 license (http://creativecommons.org/licenses/by-nc/4.0/).
Download article (PDF)
View full text (HTML)
Cite this article
TY - JOUR AU - Sultan Daud Khan AU - Saleh Basalamah PY - 2021 DA - 2021/03/30 TI - Multi-Scale Person Localization With Multi-Stage Deep Sequential Framework JO - International Journal of Computational Intelligence Systems SP - 1217 EP - 1228 VL - 14 IS - 1 SN - 1875-6883 UR - https://doi.org/10.2991/ijcis.d.210326.001 DO - 10.2991/ijcis.d.210326.001 ID - Khan2021 ER -