A Comprehensive Study of Machine Learning Methods on Diabetic Retinopathy Classification
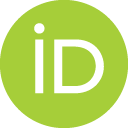
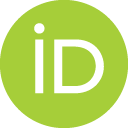
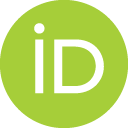
- DOI
- 10.2991/ijcis.d.210316.001How to use a DOI?
- Keywords
- machine learning; ensemble learning; transfer learning; XGBoost; PCA; SVD
- Abstract
Diabetes is one of the emerging threats to public health all over the world. According to projections by the World Health Organization, diabetes will be the seventh foremost cause of death in 2030 (WHO, Diabetes, 2020. https://www.afro.who.int/health-topics/diabetes). Diabetic retinopathy (DR) results from long-lasting diabetes and is the fifth leading cause of visual impairment, worldwide. Early diagnosis and treatment processes are critical to overcoming this disease. The diagnostic procedure is challenging, especially in low-resource settings, or time-consuming, depending on the ophthalmologist's experience. Recently, automated systems now address DR classification tasks. This study proposes an automated DR classification system based on preprocessing, feature extraction, and classification steps using deep convolutional neural network (CNN) and machine learning methods. Features are extracted from a pretrained model by the transfer learning approach. DR images are classified by several machine learning methods. XGBoost outperforms other methods. Dimensionality reduction algorithms are applied to obtain a lower-dimensional representation of extracted features. The proposed model is trained and evaluated on a publicly available dataset. Grid search and calibration are used in the analysis. This study provides researchers with performance comparisons of different machine learning methods. The proposed model offers a robust solution for detecting DR with a small number of images. We used a transfer learning approach, which differs from other studies in the literature, during the feature extraction. It provides a data-driven, cost-effective solution, which includes comprehensive preprocessing and fine-tuning processes.
- Copyright
- © 2021 The Authors. Published by Atlantis Press B.V.
- Open Access
- This is an open access article distributed under the CC BY-NC 4.0 license (http://creativecommons.org/licenses/by-nc/4.0/).
Download article (PDF)
View full text (HTML)
Cite this article
TY - JOUR AU - Omer Faruk Gurcan AU - Omer Faruk Beyca AU - Onur Dogan PY - 2021 DA - 2021/03/22 TI - A Comprehensive Study of Machine Learning Methods on Diabetic Retinopathy Classification JO - International Journal of Computational Intelligence Systems SP - 1132 EP - 1141 VL - 14 IS - 1 SN - 1875-6883 UR - https://doi.org/10.2991/ijcis.d.210316.001 DO - 10.2991/ijcis.d.210316.001 ID - Gurcan2021 ER -