Deep Learning Models Combining for Breast Cancer Histopathology Image Classification
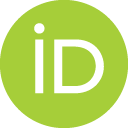
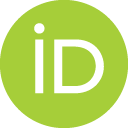
- DOI
- 10.2991/ijcis.d.210301.002How to use a DOI?
- Keywords
- Breast cancer; Histopathology images; Deep learning; Tssue malignancy; Classification
- Abstract
Breast cancer is one of the foremost reasons of death among women in the world. It has the largest mortality rate compared to the types of cancer accounting for 1.9 million per year in 2020. An early diagnosis may increase the survival rates. To this end, automating the analysis and the diagnosis allows to improve the accuracy and to reduce processing time. However, analyzing breast imagery's is non-trivial and may lead to experts' disagreements. In this research, we focus on breast cancer histopathological images acquired using the microscopic scan of breast tissues. We present combined two deep convolutional neural networks (DCNNs) to extract distinguished image features using transfer learning. The pre-trained Inception and the Xceptions models are used in parallel. Then, the feature maps are combined and reduced by dropout before being fed to the last fully connected layers for classification. We follow a sub-image classification then a whole image classification based on majority vote and maximum probability rules. Four tissue malignancy levels are considered: normal, benign, in situ carcinoma, and invasive carcinoma. The experimentations are performed to the Breast Cancer Histology (BACH) dataset. The overall accuracy for the sub-image classification is 97.29% and for the carcinoma cases the sensitivity achieved 99.58%. The whole image classification overall accuracy reaches 100% by majority vote and 95% by maximum probability fusion decision. The numerical results showed that our proposed approach outperforms the previous methods in terms of accuracy and sensitivity. The proposed design allows an extension to whole-slide histology images classification.
- Copyright
- © 2021 The Authors. Published by Atlantis Press B.V.
- Open Access
- This is an open access article distributed under the CC BY-NC 4.0 license (http://creativecommons.org/licenses/by-nc/4.0/).
Download article (PDF)
View full text (HTML)
Cite this article
TY - JOUR AU - Hela Elmannai AU - Monia Hamdi AU - Abeer AlGarni PY - 2021 DA - 2021/03/08 TI - Deep Learning Models Combining for Breast Cancer Histopathology Image Classification JO - International Journal of Computational Intelligence Systems SP - 1003 EP - 1013 VL - 14 IS - 1 SN - 1875-6883 UR - https://doi.org/10.2991/ijcis.d.210301.002 DO - 10.2991/ijcis.d.210301.002 ID - Elmannai2021 ER -