Few-Shot Image Segmentation Based on Dual Comparison Module and Sequential k-Shot Integration
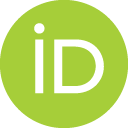
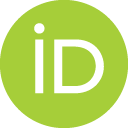
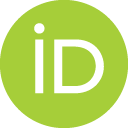
- DOI
- 10.2991/ijcis.d.210212.003How to use a DOI?
- Keywords
- Few-shot learning; Image segmentation; Dual comparison module; Convolutional-gated recurrent unit
- Abstract
Few-shot image segmentation intends to segment query images (test images) given only a few support samples with annotations. However, previous works ignore the impact of the object scales, especially in the support images. Meanwhile, current models only work on images with the similar size of the object and rarely test on other domains. This paper proposes a new few-shot segmentation model named DCNet, which fully exploits the support set images and their annotations and is able to generalize to the test images with unseen objects of various scales. The idea is to gradually compare the features from the query and the support image, and refine the features for the query. Furthermore, a sequential k-shot comparison method is proposed based on the ConvGRU to integrate features from multiple annotated support images. Experiments on Pascal VOC dataset and X-ray Security Images demonstrate the excellent generalization performance of our model.
- Copyright
- © 2021 The Authors. Published by Atlantis Press B.V.
- Open Access
- This is an open access article distributed under the CC BY-NC 4.0 license (http://creativecommons.org/licenses/by-nc/4.0/).
Download article (PDF)
View full text (HTML)
Cite this article
TY - JOUR AU - Chencong Xing AU - Shujing Lyu AU - Yue Lu PY - 2021 DA - 2021/03/01 TI - Few-Shot Image Segmentation Based on Dual Comparison Module and Sequential k-Shot Integration JO - International Journal of Computational Intelligence Systems SP - 886 EP - 895 VL - 14 IS - 1 SN - 1875-6883 UR - https://doi.org/10.2991/ijcis.d.210212.003 DO - 10.2991/ijcis.d.210212.003 ID - Xing2021 ER -