Slope Sliding Force Prediction via Belief Rule-Based Inferential Methodology
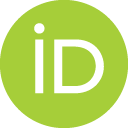
- DOI
- 10.2991/ijcis.d.210216.001How to use a DOI?
- Keywords
- Slope landslide; Sliding force; Belief rule base; SLP optimization algorithm; West–East Gas Pipeline Project
- Abstract
Slope sliding force can be measured by an anchor cable sensor with the negative Poisson's ratio (NPR) property. It is capable of reflecting the stability of the slope intuitively. Thus, predicting the variation trend of the sliding force is able to achieve early warning for landslide disaster, thereby avoiding losses to the lives and property of the people. In this paper, due to the uncertain variation of the sliding force, a belief rule-based (BRB) sliding force prediction model is established to describe the nonlinear and uncertain relationship between the history/current sliding force and the future sliding force. In this model, the activated belief rules are fused by adopting the evidence reasoning (ER) algorithm. And based on the fused results, the sliding force at a future time can be predicted accurately. Moreover, considering the variation of the sliding force on different slopes or different monitoring points in the same slope, a parameter transfer strategy of BRB model together with a corresponding online update method are proposed to achieve the adaptive design of the BRB prediction model. Finally, the effectiveness of the proposed sliding force prediction methods has been verified by experiments on the sub-section of the China West–East Gas Pipeline Project.
- Copyright
- © 2021 The Authors. Published by Atlantis Press B.V.
- Open Access
- This is an open access article distributed under the CC BY-NC 4.0 license (http://creativecommons.org/licenses/by-nc/4.0/).
Download article (PDF)
View full text (HTML)
Cite this article
TY - JOUR AU - Jing Feng AU - Xiaobin Xu AU - Pan Liu AU - Feng Ma AU - Chengrong Ma AU - Zhigang Tao PY - 2021 DA - 2021/02/25 TI - Slope Sliding Force Prediction via Belief Rule-Based Inferential Methodology JO - International Journal of Computational Intelligence Systems SP - 965 EP - 977 VL - 14 IS - 1 SN - 1875-6883 UR - https://doi.org/10.2991/ijcis.d.210216.001 DO - 10.2991/ijcis.d.210216.001 ID - Feng2021 ER -