Automatic Acute Ischemic Stroke Lesion Segmentation Using Semi-supervised Learning
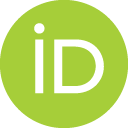
- DOI
- 10.2991/ijcis.d.210205.001How to use a DOI?
- Keywords
- Semi-supervised learning; Acute ischemic stroke lesion segmentation; Convolutional neural network (CNN); K-Means; Region growing
- Abstract
Ischemic stroke has been a common disease in the elderly population, which can cause long-term disability and even death. However, the time window for treatment of ischemic stroke in its acute stage is very short. To fast localize and quantitively evaluate the acute ischemic stroke (AIS) lesions, many deep-learning-based lesion segmentation methods have been proposed in the literature, where a deep convolutional neural network (CNN) was trained on hundreds of fully-labeled subjects with accurate annotations of AIS lesions. Such methods, however, require a large number of subjects with pixel-by-pixel labels, making it very time-consuming in data collection and annotation. Therefore, in this paper, we propose to use a large number of weakly-labeled subjects with easy-obtained slice-level labels and a few fully-labeled ones with pixel-level annotations, and propose a semi-supervised learning method. In particular, a double-path classification network (DPC-Net) was proposed and trained using the weakly-labeled subjects to detect the suspicious AIS lesions. A K-means algorithm was used on the diffusion -weighted images (DWIs) to identify the potential AIS lesions due to the a priori knowledge that the AIS lesions appear as hyperintense. Finally, a region-growing algorithm combines the outputs of the DPC-Net and the K-means to obtain the precise lesion segmentation. By using 460 weakly-labeled subjects and 5 fully-labeled subjects to train and fine-tune the proposed method, our proposed method achieves a mean dice coefficient of 0.642, and a lesion-wise F1 score of 0.822 on a clinical dataset with 150 subjects.
- Copyright
- © 2021 The Authors. Published by Atlantis Press B.V.
- Open Access
- This is an open access article distributed under the CC BY-NC 4.0 license (http://creativecommons.org/licenses/by-nc/4.0/).
Download article (PDF)
View full text (HTML)
Cite this article
TY - JOUR AU - Bin Zhao AU - Shuxue Ding AU - Hong Wu AU - Guohua Liu AU - Chen Cao AU - Song Jin AU - Zhiyang Liu PY - 2021 DA - 2021/02/10 TI - Automatic Acute Ischemic Stroke Lesion Segmentation Using Semi-supervised Learning JO - International Journal of Computational Intelligence Systems SP - 723 EP - 733 VL - 14 IS - 1 SN - 1875-6883 UR - https://doi.org/10.2991/ijcis.d.210205.001 DO - 10.2991/ijcis.d.210205.001 ID - Zhao2021 ER -