Evolutionary Multimodal Optimization Based on Bi-Population and Multi-Mutation Differential Evolution
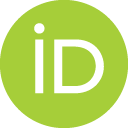
- DOI
- 10.2991/ijcis.d.200826.001How to use a DOI?
- Keywords
- Differential evolution; Multi-mutation strategy; Fitness Euclidean-distance ratio; Multimodal optimization problems
- Abstract
The most critical issue of multimodal evolutionary algorithms (EAs) is to find multiple distinct global optimal solutions in a run. EAs have been considered as suitable tools for multimodal optimization because of their population-based structure. However, EAs tend to converge toward one of the optimal solutions due to the difficulty of population diversity preservation. In this paper, we propose a bi-population and multi-mutation differential evolution (BMDE) algorithm for multimodal optimization problems. The novelties and contribution of BMDE include the following three aspects: First, bi-population evolution strategy is employed to perform multimodal optimization in parallel. The difference between inferior solutions and the current population can be considered as a promising direction toward the optimum. Second, multi-mutation strategy is introduced to balance exploration and exploitation in generating offspring. Third, the update strategy is applied to individuals with high similarity, which can improve the population diversity. Experimental results on CEC2013 benchmark problems show that the proposed BMDE algorithm is better than or at least comparable to the state-of-the-art multimodal algorithms in terms of the quantity and quality of the optimal solutions.
- Copyright
- © 2020 The Authors. Published by Atlantis Press B.V.
- Open Access
- This is an open access article distributed under the CC BY-NC 4.0 license (http://creativecommons.org/licenses/by-nc/4.0/).
Download article (PDF)
View full text (HTML)
Cite this article
TY - JOUR AU - Wei Li AU - Yaochi Fan AU - Qingzheng Xu PY - 2020 DA - 2020/09/11 TI - Evolutionary Multimodal Optimization Based on Bi-Population and Multi-Mutation Differential Evolution JO - International Journal of Computational Intelligence Systems SP - 1345 EP - 1367 VL - 13 IS - 1 SN - 1875-6883 UR - https://doi.org/10.2991/ijcis.d.200826.001 DO - 10.2991/ijcis.d.200826.001 ID - Li2020 ER -