YOLOv3: Face Detection in Complex Environments
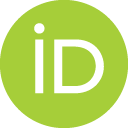
- DOI
- 10.2991/ijcis.d.200805.002How to use a DOI?
- Keywords
- Face detection; Complex environment; Priori box; Multiple score values
- Abstract
Face detection has been well studied for many years. However, the problem of face detection in complex environments is still being studied. In complex environments, faces is often blocked and blurred. This article proposes applying YOLOv3 to face detection problems in complex environments. First, we will re-cluster the data set in order to find the most suitable a priori box. Then we set multiple score values to make it possible to predict the results of multiple sets of images and find the optimal score value. Experimental results show that after adjustment, the model has more advantages in face detection than the original model in complex environments. The average accuracy is more than 10% higher than that of aggregate channel feature (ACF), Tow-stage convolutional neural network (CNN) and multi-scale Cascade CNN in face detection benchmarks WIDER FACE. Our code is available in: git@github.com:Mrtake/-complex--scenes-faceYOLOv3.git
- Copyright
- © 2020 The Authors. Published by Atlantis Press B.V.
- Open Access
- This is an open access article distributed under the CC BY-NC 4.0 license (http://creativecommons.org/licenses/by-nc/4.0/).
Download article (PDF)
View full text (HTML)
Cite this article
TY - JOUR AU - Lin Zheng Chun AU - Li Dian AU - Jiang Yun Zhi AU - Wang Jing AU - Chao Zhang PY - 2020 DA - 2020/08/17 TI - YOLOv3: Face Detection in Complex Environments JO - International Journal of Computational Intelligence Systems SP - 1153 EP - 1160 VL - 13 IS - 1 SN - 1875-6883 UR - https://doi.org/10.2991/ijcis.d.200805.002 DO - 10.2991/ijcis.d.200805.002 ID - Chun2020 ER -