Scalable Real-Time Attributes Responsive Extreme Learning Machine
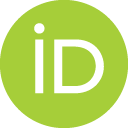
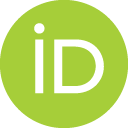
- DOI
- 10.2991/ijcis.d.200731.001How to use a DOI?
- Keywords
- Extreme learning machine; Attributes scalable; Cropping strategy
- Abstract
Extreme learning machine (ELM) has recently attracted many researchers' interest due to its very fast learning speed, and ease of implementation. Its many applications, such as regression, binary and multiclass classification, acquired better results. However, when some attributes of the dataset have been lost, this fixed network structure will be less than satisfactory. This article suggests a Scalable Real-Time Attributes Responsive Extreme Learning Machine (Star-ELM), which can grow its appropriate structure with nodes autonomous coevolution based on the different dataset. Its hidden nodes can be merged to more effectively adjust structure and weight. In the experiments of classical datasets we compare with other relevant variants of ELM, Star-ELM makes better performance on classification learning with loss of dataset attributes in some situations.
- Copyright
- © 2020 The Authors. Published by Atlantis Press B.V.
- Open Access
- This is an open access article distributed under the CC BY-NC 4.0 license (http://creativecommons.org/licenses/by-nc/4.0/).
Download article (PDF)
View full text (HTML)
Cite this article
TY - JOUR AU - Hongbo Wang AU - Yuejuan Yao AU - Xi Liu AU - Xuyan Tu PY - 2020 DA - 2020/08/10 TI - Scalable Real-Time Attributes Responsive Extreme Learning Machine JO - International Journal of Computational Intelligence Systems SP - 1101 EP - 1108 VL - 13 IS - 1 SN - 1875-6883 UR - https://doi.org/10.2991/ijcis.d.200731.001 DO - 10.2991/ijcis.d.200731.001 ID - Wang2020 ER -