Volume 13, Issue 1, 2020, Pages 717 - 726
Dose Regulation Model of Norepinephrine Based on LSTM Network and Clustering Analysis in Sepsis
Authors
Jingming Liu1, Minghui Gong2, Wei Guo1, Chunping Li2, *,
, Hui Wang3, Shuai Zhang3, Christopher Nugent3
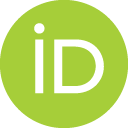
1Emergency Department in Beijing Tiantan Hospital, Capital Medical University, Beijing 100070, China
2School of Software, Tsinghua University, Beijing 100084, China
3Faculty of Computing, Ulster University Jordanstown, Northern Ireland BT37 0QB, UK
*Corresponding author. Email: cli@tsinghua.edu.cn
Corresponding Author
Chunping Li
Received 16 November 2019, Accepted 7 May 2020, Available Online 29 June 2020.
- DOI
- 10.2991/ijcis.d.200512.001How to use a DOI?
- Keywords
- Time series data; LSTM; Clustering; Sepsis; Blood pressure regulation
- Abstract
Sepsis is a life-threatening condition that arises when the body's response to infection causes injury to its own tissues and organs. Despite the advancement of medical diagnosis and treatment technologies, the morbidity and mortality of sepsis are still relatively high. In this paper, a two-layer long short-term memory (LSTM) model is proposed to predict the dose of norepinephrine, in order to control the blood pressure of patients. The proposed modeling approach is evaluated using the MIMIC-III dataset, achieving higher performance.
- Copyright
- © 2020 The Authors. Published by Atlantis Press SARL.
- Open Access
- This is an open access article distributed under the CC BY-NC 4.0 license (http://creativecommons.org/licenses/by-nc/4.0/).
Download article (PDF)
View full text (HTML)
Cite this article
TY - JOUR AU - Jingming Liu AU - Minghui Gong AU - Wei Guo AU - Chunping Li AU - Hui Wang AU - Shuai Zhang AU - Christopher Nugent PY - 2020 DA - 2020/06/29 TI - Dose Regulation Model of Norepinephrine Based on LSTM Network and Clustering Analysis in Sepsis JO - International Journal of Computational Intelligence Systems SP - 717 EP - 726 VL - 13 IS - 1 SN - 1875-6883 UR - https://doi.org/10.2991/ijcis.d.200512.001 DO - 10.2991/ijcis.d.200512.001 ID - Liu2020 ER -