An Efficient Clustering Algorithm for Mixed Dataset of Postoperative Surgical Records
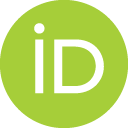
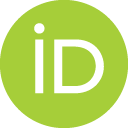
- DOI
- 10.2991/ijcis.d.200601.001How to use a DOI?
- Keywords
- Data clustering; Meta-heuristic; Artificial electric field algorithm; Distance measure; Mixed dataset
- Abstract
In data mining, data clustering is a prevalent data analysis methodology that organizes unlabeled data points into distinct clusters based on a similarity measure. In recent years, several clustering algorithms found, dependent on a predefined number of clusters and centered around the dataset with either numeric or categorical attributes only. However, many real-world engineering, scientific, and industrial applications involve datasets with mixed numeric as well as categorical attributes but lack domain knowledge (target labels). Clustering unlabeled-mixed datasets is a challenging task as (1) it is difficult to estimate the number of clusters in the absence of domain knowledge and (2) mathematical operations cannot be applied directly to the mixed dataset. In this paper, an efficient searching and fast convergent automatic data clustering algorithm based on population-based meta-heuristic optimization is proposed to deal with the mixed dataset. The proposed clustering algorithm aims to find the optimal number of cluster partitions automatically. It utilizes a real-coded variable-length candidate solution to detect the optimal number of clusters automatically. The concepts of threshold setting and cut-off ratio are used in the optimization process to refine the clusters. The similarity between data points and different cluster centers is measured using Euclidean distance (for numeric attributes) and the probability of co-occurrence of values (for categorical attributes). The proposed algorithm is compared with existing mixed data clustering techniques based on a statistical significance test and two robustness measures: Average accuracy and Standard deviation. Finally, the proposed algorithm is validated by applying to a real historical postoperative surgical mixed data set obtained from a surgical department of a multispecialty hospital in India. Results show the effectiveness, robustness, and usefulness of the proposed clustering algorithm.
- Copyright
- © 2020 The Authors. Published by Atlantis Press SARL.
- Open Access
- This is an open access article distributed under the CC BY-NC 4.0 license (http://creativecommons.org/licenses/by-nc/4.0/).
Download article (PDF)
View full text (HTML)
Cite this article
TY - JOUR AU - Hemant Petwal AU - Rinkle Rani PY - 2020 DA - 2020/06/18 TI - An Efficient Clustering Algorithm for Mixed Dataset of Postoperative Surgical Records JO - International Journal of Computational Intelligence Systems SP - 757 EP - 770 VL - 13 IS - 1 SN - 1875-6883 UR - https://doi.org/10.2991/ijcis.d.200601.001 DO - 10.2991/ijcis.d.200601.001 ID - Petwal2020 ER -