Weighted Nonnegative Matrix Factorization for Image Inpainting and Clustering
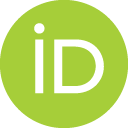
- DOI
- 10.2991/ijcis.d.200527.003How to use a DOI?
- Keywords
- Recovery; Dimensionality reduction; Weighted nonnegative matrix factorization; Noise
- Abstract
Conventional nonnegative matrix factorization and its variants cannot separate the noise data space into a clean space and learn an effective low-dimensional subspace from Salt and Pepper noise or Contiguous Occlusion. This paper proposes a weighted nonnegative matrix factorization (WNMF) to improve the robustness of existing nonnegative matrix factorization. In WNMF, a weighted graph is constructed to label the uncorrupted data as 1 and the corrupted data as 0, and an effective matrix factorization model is proposed to recover the noise data and achieve clustering from the recovered data. Extensive experiments on the image datasets corrupted by Salt and Pepper noise or Contiguous Occlusion are presented to demonstrate the effectiveness and robustness of the proposed method in image inpainting and clustering.
- Copyright
- © 2020 The Authors. Published by Atlantis Press SARL.
- Open Access
- This is an open access article distributed under the CC BY-NC 4.0 license (http://creativecommons.org/licenses/by-nc/4.0/).
Download article (PDF)
View full text (HTML)
Cite this article
TY - JOUR AU - Xiangguang Dai AU - Nian Zhang AU - Keke Zhang AU - Jiang Xiong PY - 2020 DA - 2020/06/17 TI - Weighted Nonnegative Matrix Factorization for Image Inpainting and Clustering JO - International Journal of Computational Intelligence Systems SP - 734 EP - 743 VL - 13 IS - 1 SN - 1875-6883 UR - https://doi.org/10.2991/ijcis.d.200527.003 DO - 10.2991/ijcis.d.200527.003 ID - Dai2020 ER -