Blur2Sharp: A GAN-Based Model for Document Image Deblurring
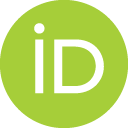
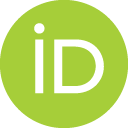
- DOI
- 10.2991/ijcis.d.210407.001How to use a DOI?
- Keywords
- Generative adversarial network (GAN); Cycle-consistent generative adversarial network (CycleGAN); Document deblurring; Blind deconvolution; Motion blur; Out-of-focus blur
- Abstract
The advances in mobile technology and portable cameras have facilitated enormously the acquisition of text images. However, the blur caused by camera shake or out-of-focus problems may affect the quality of acquired images and their use as input for optical character recognition (OCR) or other types of document processing. This work proposes an end-to-end model for document deblurring using cycle-consistent adversarial networks. The main novelty of this work is to achieve blind document deblurring, i.e., deblurring without knowledge of the blur kernel. Our method, named “Blur2Sharp CycleGAN,” generates a sharp image from a blurry one and shows how cycle-consistent generative adversarial networks (CycleGAN) can be used in document deblurring. Using only a blurred image as input, we try to generate the sharp image. Thus, no information about the blur kernel is required. In the evaluation part, we use peak signal to noise ratio (PSNR) and structural similarity index (SSIM) to compare the deblurring images. The experiments demonstrate a clear improvement in visual quality with respect to the state-of-the-art using a dataset of text images.
- Copyright
- © 2021 The Authors. Published by Atlantis Press B.V.
- Open Access
- This is an open access article distributed under the CC BY-NC 4.0 license (http://creativecommons.org/licenses/by-nc/4.0/).
Download article (PDF)
View full text (HTML)
Cite this article
TY - JOUR AU - Hala Neji AU - Mohamed Ben Halima AU - Tarek M. Hamdani AU - Javier Nogueras-Iso AU - Adel M. Alimi PY - 2021 DA - 2021/04/13 TI - Blur2Sharp: A GAN-Based Model for Document Image Deblurring JO - International Journal of Computational Intelligence Systems SP - 1315 EP - 1321 VL - 14 IS - 1 SN - 1875-6883 UR - https://doi.org/10.2991/ijcis.d.210407.001 DO - 10.2991/ijcis.d.210407.001 ID - Neji2021 ER -