Neural Dialogue Generation Methods in Open Domain: A Survey
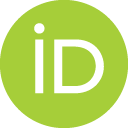
- DOI
- 10.2991/nlpr.d.210223.001How to use a DOI?
- Keywords
- Neural dialogue generation; Open-domain dialogue system; Natural language processing
- Abstract
Open-Domain Dialogue Generation (human–computer interaction) is an important issue in the field of Natural Language Processing (NLP). Because of the improvement of deep learning techniques, a large number of neural dialogue generative methods were proposed to generate better responses. In this survey, we elaborated the research history of these existing generative methods, and then roughly divided them into six categories, i.e., Encoder-Decoder framework-based methods, Hierarchical Recurrent Encoder-Decoder (HRED)-based methods, Variational Autoencoder (VAE)-based methods, Reinforcement Learning (RL)- based methods, Generative Adversarial Network (GAN)-based methods, and pretraining-model-based methods. We dived into the methods of each category and gave the detailed discussions of these methods. After that, we presented a comparison among the different categories of methods and analyzed their advantages and disadvantages. We enumerated some open access public datasets and some commonly used automatic evaluating metrics. Finally, we discuss some possible research directions that can take the research of neural dialogue generation into a new frontier in the future.
- Copyright
- © 2021 The Authors. Published by Atlantis Press B.V.
- Open Access
- This is an open access article under the CC BY-NC license (http://creativecommons.org/licenses/by-nc/4.0/).
Download article (PDF)
View full text (HTML)
Cite this article
TY - JOUR AU - Bin Sun AU - Kan Li PY - 2021 DA - 2021/03/19 TI - Neural Dialogue Generation Methods in Open Domain: A Survey JO - Natural Language Processing Research SP - 56 EP - 70 VL - 1 IS - 3-4 SN - 2666-0512 UR - https://doi.org/10.2991/nlpr.d.210223.001 DO - 10.2991/nlpr.d.210223.001 ID - Sun2021 ER -