Forecasting Teleconsultation Demand with an Ensemble Attention-Based Bidirectional Long Short-Term Memory Model
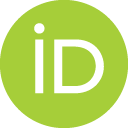
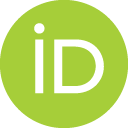
- DOI
- 10.2991/ijcis.d.210203.004How to use a DOI?
- Keywords
- Teleconsultation; Demand forecast; Holiday effect; Attention mechanism; Deep learning ensemble; Bidirectional long short-term memory (BILSTM)
- Abstract
Accurate demand forecast can help improve teleconsultation efficiency. But teleconsultation demand forecast has not been reported in existing literature. For this purpose, the study proposes a novel model based on deep learning algorithm for daily teleconsultation demand forecast to fill in the research gap. Because of the significant effect of holidays on teleconsultation demand, holiday-related variables, and specific prediction technologies were selected to treat it. The technologies attention mechanism and bidirectional long short-term memory (BILSTM) were used to construct a novel forecasting methodology, i.e., ensemble attention-based BILSTM (EA-BILSTM), for the accurate forecasts. Based on actual teleconsultation data, the effectiveness of variable selection is verified by importing different inputs into models, and the superiority of EA-BILSTM is verified by comparison with nine benchmark models. Empirical results show that importing selected variables can lead to better forecasts and EA-BILSTM model can get lowest forecasting errors on two sub-datasets. This indicates that the proposed forecasting model is a high potential approach for teleconsultation demand prediction in the influence of sparse trait, like holiday effects.
- Copyright
- © 2021 The Authors. Published by Atlantis Press B.V.
- Open Access
- This is an open access article distributed under the CC BY-NC 4.0 license (http://creativecommons.org/licenses/by-nc/4.0/).
Download article (PDF)
View full text (HTML)
Cite this article
TY - JOUR AU - Wenjia Chen AU - Lean Yu AU - Jinlin Li PY - 2021 DA - 2021/02/10 TI - Forecasting Teleconsultation Demand with an Ensemble Attention-Based Bidirectional Long Short-Term Memory Model JO - International Journal of Computational Intelligence Systems SP - 821 EP - 833 VL - 14 IS - 1 SN - 1875-6883 UR - https://doi.org/10.2991/ijcis.d.210203.004 DO - 10.2991/ijcis.d.210203.004 ID - Chen2021 ER -