Dual Neural Network Fusion Model for Chinese Named Entity Recognition
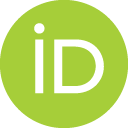
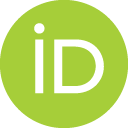
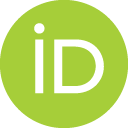
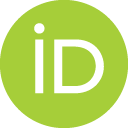
- DOI
- 10.2991/ijcis.d.201216.001How to use a DOI?
- Keywords
- Chinese named entity recognition; Dual neural network fusion; Bi-directional long-short-term memory; Self-attention mechanism; Dilated convolutional neural network
- Abstract
Chinese named entity recognition (NER) has important effect on natural language processing (NLP) applications. This recognition task is complicated in its strong dependent-relation, missing delimiters in the text and insufficient feature representation in a single model. This paper thus proposes a dual neural network fusion model (DFM) to improve Chinese NER performance. We integrate the traditional bi-directional long-short-term memory (BiLSTM) structure and self-attention mechanism (ATT) with dilated convolutional neural network (DCNN) to better capture context information. Additionally, we exploit the Google's pretrained model named bi-directional encoder representations from transformers (BERT) as the embedding layer. The proposed model has the following merits: (1) a dual neural network architecture is proposed to enhance the robustness of extracted features. (2) An attention mechanism is fused into the dual neural network to extract implicit context representation information in Chinese NER. (3) Dilated convolutions are used to make a tradeoff between performance and executing speed. Experiments show that our proposed model exceeds the state-of-the-art Chinese NER methods.
- Copyright
- © 2021 The Authors. Published by Atlantis Press B.V.
- Open Access
- This is an open access article distributed under the CC BY-NC 4.0 license (http://creativecommons.org/licenses/by-nc/4.0/).
Download article (PDF)
View full text (HTML)
Cite this article
TY - JOUR AU - Dandan Zhao AU - Jingxiang Cao AU - Degen Huang AU - Jiana Meng AU - Pan Zhang PY - 2020 DA - 2020/12/24 TI - Dual Neural Network Fusion Model for Chinese Named Entity Recognition JO - International Journal of Computational Intelligence Systems SP - 471 EP - 481 VL - 14 IS - 1 SN - 1875-6883 UR - https://doi.org/10.2991/ijcis.d.201216.001 DO - 10.2991/ijcis.d.201216.001 ID - Zhao2020 ER -