Deep Learning-Based Short-Term Load Forecasting for Transformers in Distribution Grid
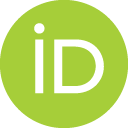
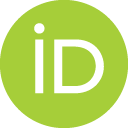
- DOI
- 10.2991/ijcis.d.201027.001How to use a DOI?
- Keywords
- Load forecasting; convolutional neural network (CNN); Long short-term memory (LSTM); Inception structure; Residual connection
- Abstract
Load of transformer in distribution grid fluctuates according to many factors, resulting in overload frequently which affects the safety of power grid. And short-term load forecasting is considered. To improve forecasting accuracy, the input information and the model structure are both considered. First, the multi-dimensional information containing numerical data and textual data is taken as the inputs of constructed deep learning model, and textual data is encoded by one-hot method. Then, for the purpose of mining the features of data better, based on the framework composed of convolutional neural network (CNN) and long short-term memory (LSTM), the modified inception structure is introduced to extract more detailed features and adaptive residual connection is added to settle the problem of gradient diffusion when the layers of model grow more. At last, the comparison is carried out and the improvements are presented after the textual data is added and the structure of model is modified. And the forecasting error is reduced, especially when the load is heavy, which is beneficial for the prevention of overload of transformer in distribution gird.
- Copyright
- © 2021 The Authors. Published by Atlantis Press B.V.
- Open Access
- This is an open access article distributed under the CC BY-NC 4.0 license (http://creativecommons.org/licenses/by-nc/4.0/).
Download article (PDF)
View full text (HTML)
Cite this article
TY - JOUR AU - Renshu Wang AU - Jing Zhao PY - 2020 DA - 2020/11/03 TI - Deep Learning-Based Short-Term Load Forecasting for Transformers in Distribution Grid JO - International Journal of Computational Intelligence Systems SP - 1 EP - 10 VL - 14 IS - 1 SN - 1875-6883 UR - https://doi.org/10.2991/ijcis.d.201027.001 DO - 10.2991/ijcis.d.201027.001 ID - Wang2020 ER -